GraphOracle: A Foundation Model for Universal Knowledge Graph Reasoning
Mar 25, 2025ยท
,ยท
1 min read

Enjun Du
Yongqi Zhang*
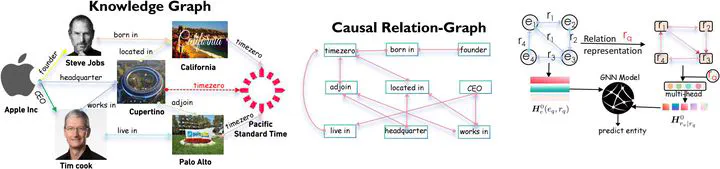
Abstract
Foundation models have demonstrated remarkable capabilities for general representation and reasoning in computer vision and natural language processing. However, developing analogous foundation models for knowledge graphs presents unique challenges due to their dynamic nature, with entities and relations requiring continuous updates. Furthermore, a significant gap exists in current models ability to perform cross-domain reasoning, particularly for critical applications in biomedical knowledge graphs. We present \textbf{GraphOracle}, a novel foundation model for universal knowledge graph reasoning that addresses these limitations. GraphOracle achieves domain-independent representations by modeling causal graphs of relationship interactions, enabling extension to arbitrary graph structures and rapid adaptation through fine-tuning. By integrating multiple unimodal foundation models, GraphOracle demonstrates robust cross-domain reasoning capabilities that extend seamlessly to biomedical knowledge graphs. Extensive experiments across Transductive, Inductive, and BioKG benchmarks demonstrate that GraphOracle consistently outperforms existing approaches and can surpass state-of-the-art models with minimal fine-tuning. Our work represents a significant advance in universal knowledge graph reasoning with important implications for biomedical discovery and cross-domain applications.
Type
Click the Cite button above to demo the feature to enable visitors to import publication metadata into their reference management software.